The predictive power of machine learning
Machine learning uses data, usually in large quantities, and statistical algorithms to predict something that isn’t known. The first article of our Driven By Data series explores how machine-learning models inform Educate Girls’ expansion strategy.
© Cartier Philanthropy / Cyril le Tourneur
In India, more than 4 million girls aged between seven and fourteen fail to show up at school every day. That’s one of the highest out-of-school rates in the world.
Educate Girls has been bridging the gender and literacy gap in the rural, most remote villages of India since 2007. In the states of Rajasthan, Madhya Pradesh and Uttar Pradesh this NGO brings girls who have dropped out of, or never enrolled in, school back to mainstream education.
Working in partnership with the Indian Government and schools, Educate Girls has always engaged with a huge base of community volunteers called Team Balika to conduct door-to-door censuses across thousands of villages in entire administrative districts. Team Balika’s main role is to challenge the ingrained mindset that prevents so many girls from becoming educated.
Household visits and community outreach are at the heart of the organisation’s success. However, the process has proven time consuming and costly over the years, making the organisation’s target of getting 1.5 million girls back to school by 2024 an immense challenge.
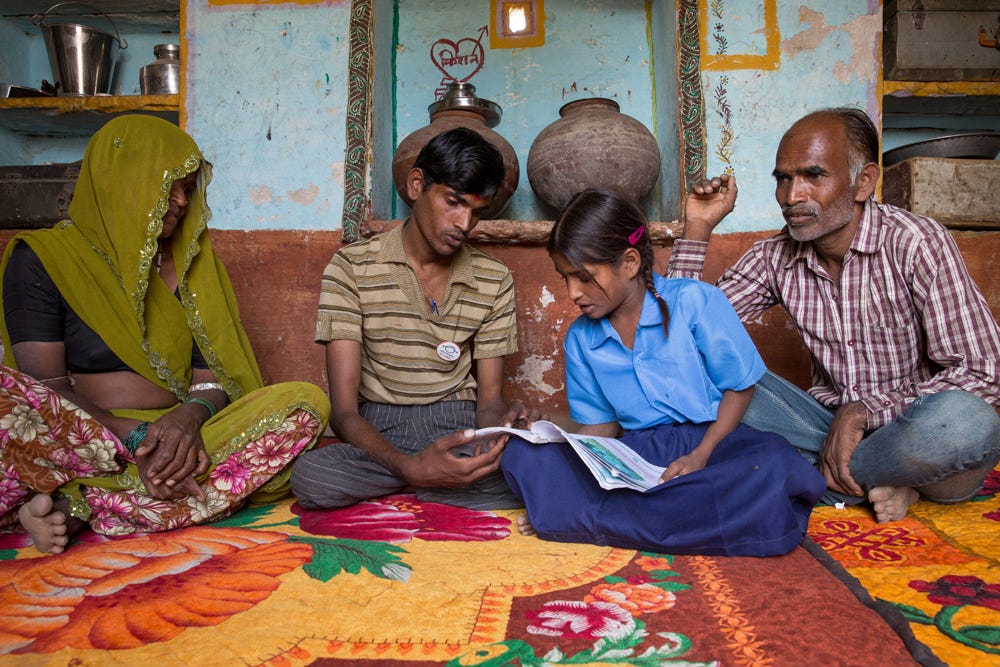
© Cartier Philanthropy / Cyril le Tourneur
The question consequently became how to prioritise villages to maximise the number of out-of-school girls reached within a limited time and budget.
That’s when predictive machine learning techniques entered the picture. Machine learning uses data, usually in large quantities, and statistical algorithms to predict something that isn’t known. It’s how navigation apps use traffic data to predict the duration of a journey or how your local supermarket knows how many loaves of bread to stock. With the help of IDinsight , Educate Girls now uses machine learning algorithms to learn from their previous Team Balika censuses how to identify villages in new geographies where they can expect to find the most out of school girls.
While rolling out its programme to the first 8,000 villages, Educate Girls had collected the enrolment status for girls from more than 1 million households. IDinsight merged this data with publicly available census and education data, as well as survey data collected by other NGOs. They then built and tested various machine learning models to predict the number of out-of-school girls in villages in other parts of the country.
Next, using these predictions, IDinsight identified geographic clusters of villages with the highest density of out of school girls. By deploying their field coordinators to these clusters, Educate Girls would find the most out of school girls who they could reach with their programme. The result? Concentrating their resources on these clusters of villages, rather than working in all the villages in a given administrative district, has enabled Educate Girls to enrol more than two and a half times the number of out-of-school girls per village as before with similar operational costs.
As the organisation expands to new geographies, the prediction algorithm continues to be updated and used to inform further expansions, making the target of 1.5 million enrolled girls by 2024 a reality.
“Since the algorithms were first created in 2018, they have been refined every year to increase in precision - new data sources, and data for more villages have been added. We will be using the recommendations of this algorithm to reach over 200,000 out-of-school children in the coming months. The model is also linked to an application that allows us to interactively create geographically proximate clusters of 8-10 villages. This enables us to precisely identify where we need to hire our local field staff and also helps minimise travel time. The use of machine learning and artificial Intelligence has been a key lever for us to maximise our reach,” explains, Lisa Rodricks, Educate Girls Vice President of Operations, Educate Girls.
Well-known for pioneering the world’s first Development Impact Bond in education, and as the first Asian non-profit to become an Audacious Project, Educate Girls has improved learning outcomes for over 1.3 million children.